What is Explainable AI and What Does it Mean for You?
As businesses expand their use of Artificial Intelligence (AI) to streamline their operations and individuals further adopt AI tools into their daily lives, it becomes necessary to understand how AI is making decisions. Explainable Artificial Intelligence (XAI) allows humans to understand AI systems’ rationale and decision-making process, which can help build trust. Conventional AI systems are pretty advanced and capable of complex tasks, but the decision-making processes are often hidden from users and stakeholders.
The lack of visibility can cause distrust as people cannot understand how the system works or why it has made certain decisions. AI surrounds us in our daily lives; it’s no longer a concept of the future. AI has also infiltrated our economy, potentially contributing $13 trillion more economic output by 2030. This article will discuss why AI explainability is necessary and what it means for you.
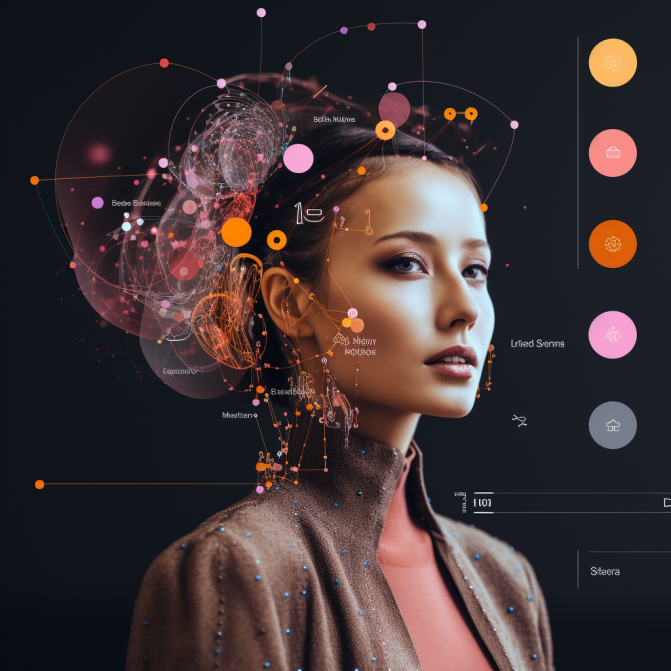
What is Explainable Artificial Intelligence?
Explainable AI is a form of AI designed to explain why it made its own decisions. Instead of just an output, XAI provides interactive explanations of how it works, and it comes to its conclusions. This helps AI practitioners understand the rationale behind AI systems and boosts trust in their decisions.
An XAI model uses natural language processing and knowledge graphs to explain how decisions were made, which are used to compare model predictions and performance in various fields, from healthcare to finance. XAI algorithms are combined with traditional AI models to uncover the logic; this is an essential step towards responsible AI, which helps increase artificial intelligence transparency.
So, what does all this mean for you? XAI can create a more transparent and accountable world, and you can trust your AI systems to make fair and responsible decisions. Transparency makes it easier for organizations to introduce autonomous systems into their operations as they can explain the rationale behind their choices. It also allows individuals to confidently use AI tools, knowing the system is trustworthy.
How Does Explainable AI Work?
XAI enables the responsible use of AI by explaining its decisions, analyzing fairness/discrimination issues, and providing greater clarity. At a basic level, Explainable AI systems use NLP and knowledge graphs to optimize model performance and break down the complex model into more digestible concepts. Not for lack of trying, but your average person is unlikely to understand neural information processing systems. Instead, let’s discuss what’s included in an Explainable AI system.
What Comprises the AI System in Explainable AI?
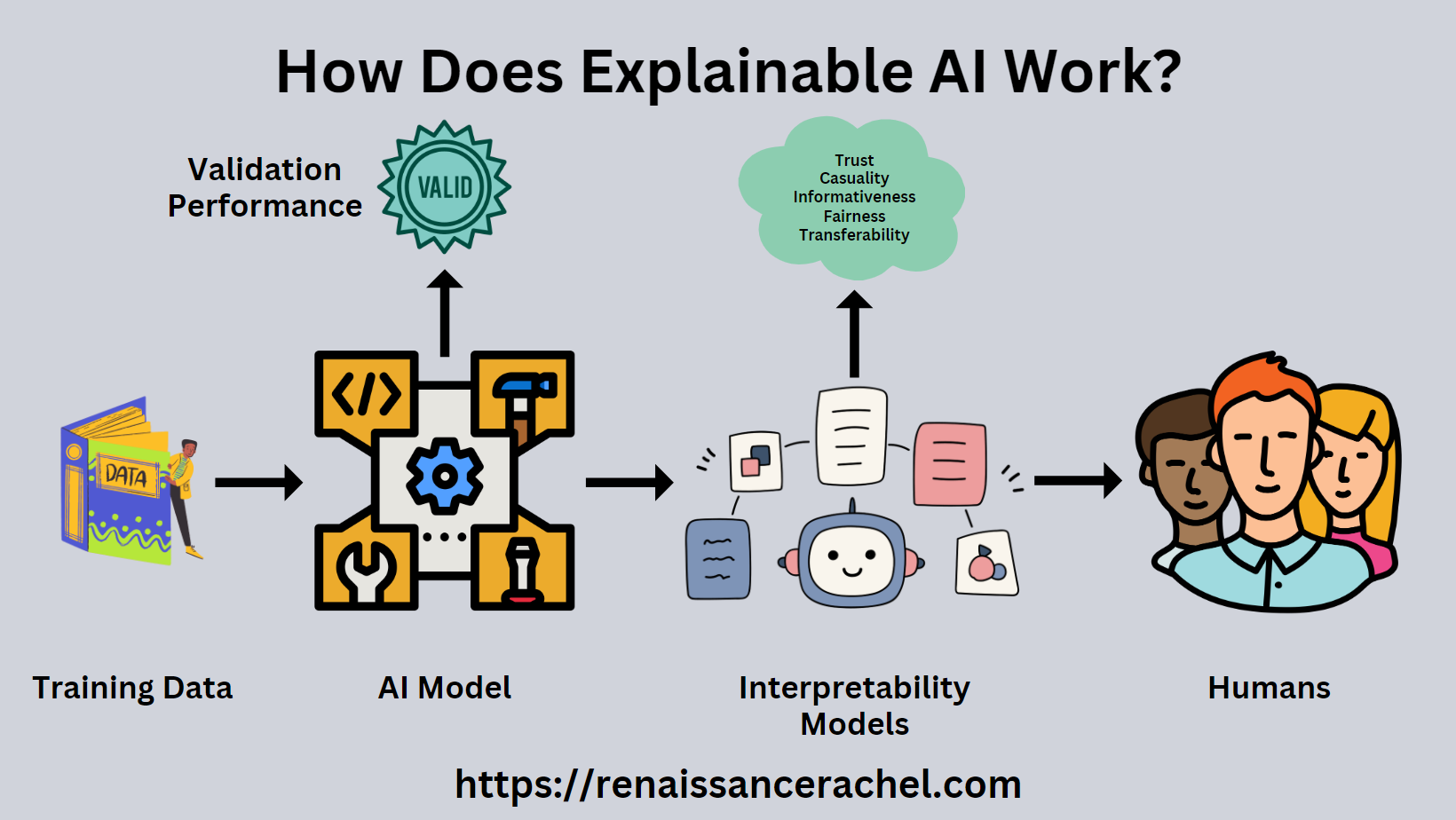
An Explainable AI system includes four main components: the Data, AI models, Interpretability Concepts, and Human Users. Interpretability concepts are a set of rules and algorithms that show the decision-making process of the AI model. In other words, the concepts of interpretability help anyone understand what the models are learning and what they can offer you.
The Data
The data component in Explainable AI refers to the vast information these AI systems interpret. This data includes anything from customer behavior patterns to complex business metrics. The key here is that Explainable AI doesn’t just crunch numbers; it provides insights about why specific recommendations were made based on the data.
It’s like having a super-smart analyst who doesn’t just hand over a spreadsheet bud walks you through the data, highlighting significant trends. This makes it easier for businesses and individuals to understand and trust the AI’s output, leading to more effective decision-making. The most achievable category of XAI is “explainable” data.
The AI Models
The AI algorithm component in Explainable AI is the system’s brain; it’s where all the decision-making takes place. These models learn from data, identify patterns, and make decisions. But here’s where Explainable AI stands out: instead of being a “black box” that spits out results, Explainable AI models allow us to visually investigate model behavior.
It gives you a peek under the hood to understand how the AI arrived at a conclusion. This transparency builds trust, as businesses and individuals can verify the AI is making sensible decisions. Explainable AI systems could be helpful in scenarios where accountability is essential, such as with a self-driving car.
This brings us back to the point: Explainable AI can revolutionize our relationship with technology and increase trust in businesses and individuals, but it doesn’t absolve us of our responsibility. Explainable AI adopts techniques that mimic human decision-making processes to enhance its interpretability.
Interpretability Concepts
Decision makers want to know how predictions are made before they can deploy AI. Interpretability concepts help explain the AI models’ inner workings, enabling humans to understand them. But that’s not all; Explainable AI doesn’t just help us understand why models do something, but who’s accountable for it. Explainable AI models are trained by incorporating explainability techniques that use simple textual descriptions to clarify the behavior.
XAI systems mimic human decision-making processes to enhance our understanding of the underlying models. While the concepts used to train data differ, we can use examples to break down the idea. A simple example of an interpretability concept might be a rule that specifies how to assess insurance claims with insufficient data.
The concepts are algorithms like a decision tree, Bayesian Networks, LIME, and Game Theory. If we break the AI techniques down further, then we get five properties:
- Causality – explores cause and effect relationships between variables.
- Trust – allows us to trust the AI model and its decision.
- Informativeness – helps us understand the model by providing insights into its decision-making process.
- Transferability – helps the AI model transfer its knowledge to new domains.
- Fairness – considers fairness aspects like racial, gender, or age biases.
The Human Users
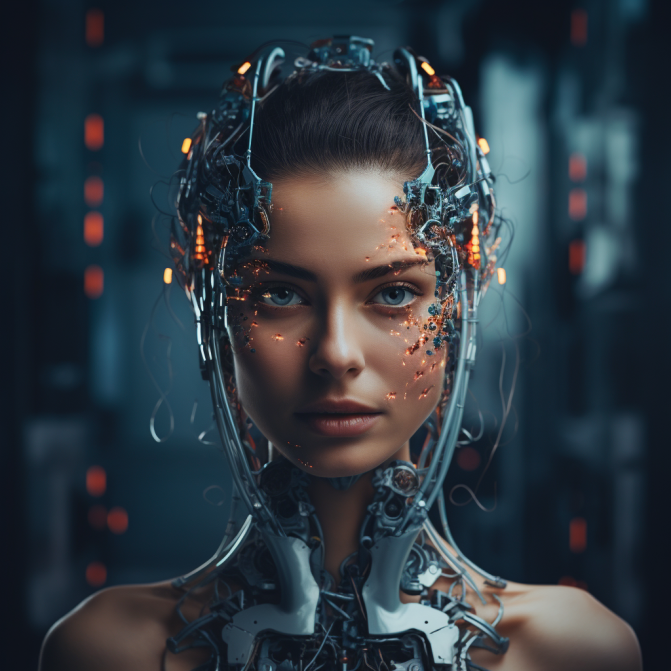
No AI system is complete without human factors. XAI is designed to bring transparency and accountability to AI systems for the benefit of humans. It’s not just about understanding the data and algorithms but trusting that the system is doing what it’s designed to do.
Still, there’s a gap between understanding and trust; we can only trust the reasonable outcome of a system if we understand its principles. XAI systems help bridge this gap by clearly explaining how a system’s decisions are regulated. To explain the rationale, let’s expand on the XAI principles.
Explainable AI Principles
We mentioned earlier that humans use interpretability concepts to understand XAI. In turn, the AI uses training data and this mandate to explain itself—to make a case for the end user. But what do these concepts mean in practice?
AI Models Should Provide Evidence
One of the fundamental principles of XAI is that artificial intelligence should provide supporting evidence for its decisions. Organizations can’t rely on purely experimental AI, scattered pilots, or build isolated AI systems in silos. Why does this matter?
Imagine you’re using a GPS. If it tells you to take a particular route, you’d want to know why. Is it the fastest route or the safest? The same principle applies to AI. When an AI model makes a decision, it’s vital that it can explain.
This evidence helps users understand the ‘why’ behind the decision, creating trust and transparency. It also allows us to verify the finding, ensuring it’s fair, unbiased, and accurate. So, providing evidence doesn’t just improve model performance; it supports proper model governance.
Accurate Explanations of Model Behavior
The principle of providing post hoc explanations of model behavior is a cornerstone of XAI. Think of it this way: an AI system is like a new team member. If this team member makes a decision or recommendation, you’d want to understand their thought process. Similarly, when an AI model analyzes data and generates an outcome, it must clearly explain its conclusion.
Transparency helps users understand the ‘why’ behind the AI’s decisions, fostering trust and enabling more informed decision-making. More importantly, accurate explanations allow for identifying and correcting any potential issues or biases in the training data. It’s not just about understanding what the system did but ensuring what it did was correct and fair.
An AI System Should Provide “Meaningful” Explanations
Providing “meaningful” explanations is a fundamental aspect of Explainable AI. But what makes an explanation ‘meaningful’? Essentially, it’s about delivering accurate, understandable, and practical insights to the user. For instance, an AI model uses a complex algorithm to decide. The intricacies of this algorithm might be too technical for most users.
A meaningful explanation, in contrast, would translate this complexity into something more digestible that the end user can use to inform their decisions. Moreover, these explanations should be tailored to the user’s context, answering their questions. In essence, meaningful explanations make AI more than a tool; they transform it into artificially intelligent machine partners that help users navigate complex decisions with confidence and clarity.
The System Should Operate Within Knowledge Limits
The principle of operating within knowledge limits is crucial for Explainable AI. Let’s break it down: AI systems have limitations, just like humans. They can only perform based on the data they’ve been trained on and the algorithms they’ve been programmed with. If AI makes a decision outside these limits, it can lead to inaccurate or unreliable results. So, we need to recognize and respect AI systems’ knowledge boundaries.
This doesn’t mean that AI models can’t adapt – on the contrary, learning is a critical part of AI. But this learning should be guided and monitored, ensuring the AI stays within its knowledge limits. This principle helps maintain the accuracy and reliability of AI systems. It also fosters trust among users because the AI’s decisions are based on proven knowledge.
Why is Explainable AI Important to Everyone?
Asking anyone to trust an autonomous workflow from the outset is a massive leap of faith. XAI is the answer to this problem: it enables users to peel back the layers and reveal interpretable machine learning. It also achieves accountability and fairness, increasing transparency and visibility, but we’re just generalizing here. Explainable AI is important, but there are many reasons why it matters.
The Difference Between Black Box vs. White Box Models
The “black box” sounds so sinister, doesn’t it? It’s an AI system that works but is too complex to explain. This makes it hard for people to trust and verify its outputs. What if it made a mistake? How would we even know when it succeeds?
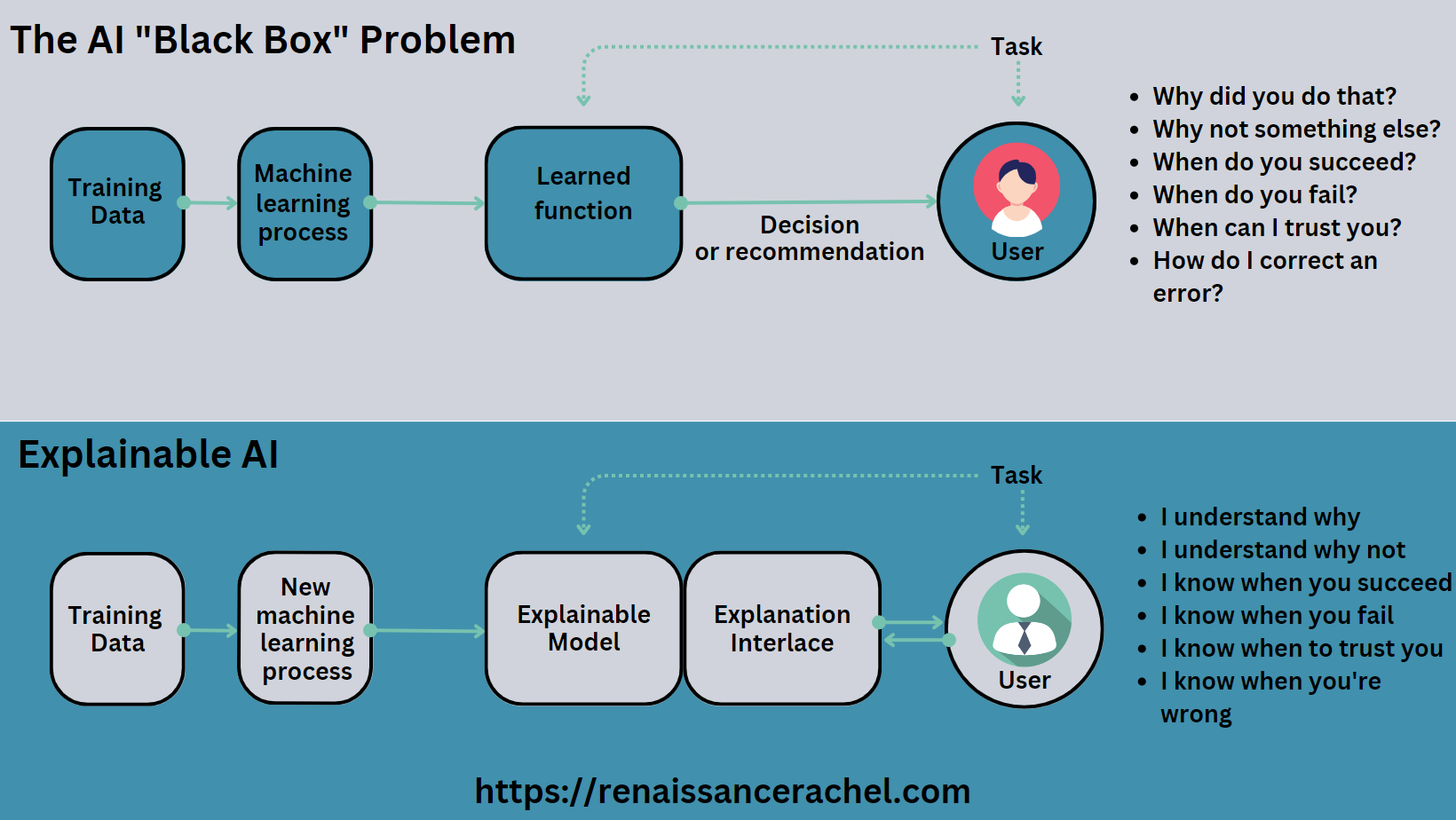
The primary difference between black box models and explainable white box AI is that the latter provides insights into how a model makes decisions. Using the above image, you can see that white box models reveal their innerworkings in a relevant manner. That’s why Explainable AI is so important: to bridge the trust gap between us using AI and those developing it.
Artificial Intelligence is Used in Sensitive Areas
The application of AI in sensitive areas such as healthcare, legal systems, warfare, and financial services is becoming increasingly prevalent. In war, advanced AI drones can take down individuals without human intervention. Although some military forces possess such technology, they remain wary of unpredictable outcomes.
They hesitate to rely on systems whose operation they cannot fully understand. Self-driving cars, like a Tesla on autopilot, provide comfort for drivers but is a substantial responsibility. We must understand how the car would handle a moral dilemma. As time goes on, AI systems are increasingly impacting our social lives.
Neural Networks and AI Algorithms Making Automated Decisions Need Trust
Neural Networks and AI are already making automated decisions, but you might wonder why machine learning algorithms are problematic. For centuries, mathematicians have worked on traditional machine learning algorithms. These algorithms were created long before computers and are very intuitive.
When you make decisions using one of these traditional algorithms, it’s easy to provide explanations. However, they only achieve a certain level of prediction accuracy. So, while our standard algorithms have high AI explainability, they have only had limited success. Limited success doesn’t work well in healthcare, finance, or law.
Since then, machine learning has undergone a revolutionary change, leading to the emergence of Deep Learning. This sub-domain of AI applications seeks to imitate the functions of our brains through Artificial Neural Networks. With superior computing power and advanced open-source deep learning frameworks, we can develop intricate networks that deliver top-notch precision.
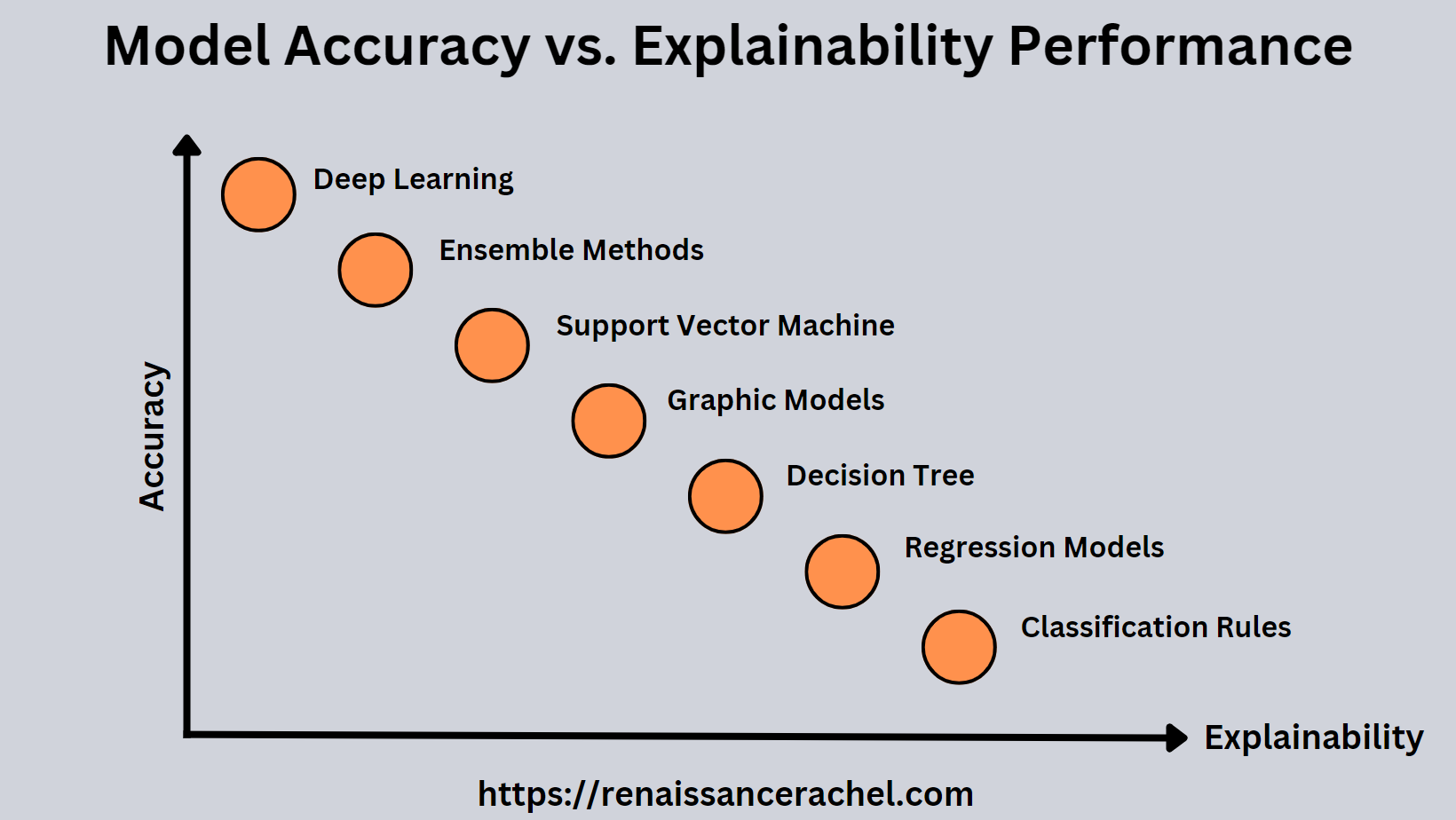
Unfortunately, neural systems are hard to understand and trained with billions of variables, hence GPT-3, GPT-4, and so on. The image above depicts the correlation between the accuracy and explainability of machine learning algorithms. It shows that developers rely on deep learning models instead of traditional algorithms in favor of accuracy. As a result, the explainability of the models is decreasing; however, we need explainability more than ever.
AI-related Disputes That Need Resolution Must Have Explanations
Regulations demand explainability due to human rights and freedoms advancements in the last two centuries. Legal argumentation and reasoning explore the limits of explainability. Despite AI taking over certain occupations, we are still responsible for providing explanations and must follow the same rules as traditional methods.
In addition, mandatory explainability is also required by current laws and regulations. For example, the General Data Protection Regulation (GDPR) in the European Union provides a “right to explanation.” GDPR requires an explanation of the logic behind AI when automated decisions affect citizens.
Removing Biases from the AI Model Itself Requires Explainability
We already know that humans are historically prejudiced; we are trying to remove biases in our society, but AI models can have them baked in if they’re not built from scrubbed data. If a developer trains an AI model using discriminatory historical data, the model could produce biased outcomes.
Let’s say a group of developers trained their AI system with biased data on Native Americans; the model would produce outcomes based on discriminatory practices. It would be devastating to Native Americans as it would promote unjust treatment. The effects can be transferred to other minority groups as well.
Machine Learning Models Could Create Threats
We’ve all heard of the devastation AI could wreak on the world – think about any movie about AI in the last 30 years. And frankly, they’re not too far off the mark. Machine learning models could create serious threats to society if left unchecked, and nearly half of 731 leading AI researchers believe that human-level AI would lead to catastrophic outcomes.
Now that it’s apparent why Explainable AI is important let’s talk about how it benefits everyone.
What are the Benefits of Explainable AI?
Explainable AI has several benefits, which include making informed decisions, decreasing risks, enhancing governance, enabling quicker system improvement, and advancing the emerging generation of AI globally.
Improves Model Performance
Explainable AI can significantly enhance model performance by providing interpretability. Think of it like having a backstage pass to a concert – seeing how everything comes together behind the scenes. In the case of AI, you can understand how the model presents outcomes.
This understanding allows developers to fine-tune the model, tweak parameters, or address accuracy issues. By doing so, the model can perform, making more accurate decisions. Plus, when users understand why a model makes specific recommendations, they will trust and use it, enhancing its predictive power and effectiveness.
It Makes AI More Trustworthy
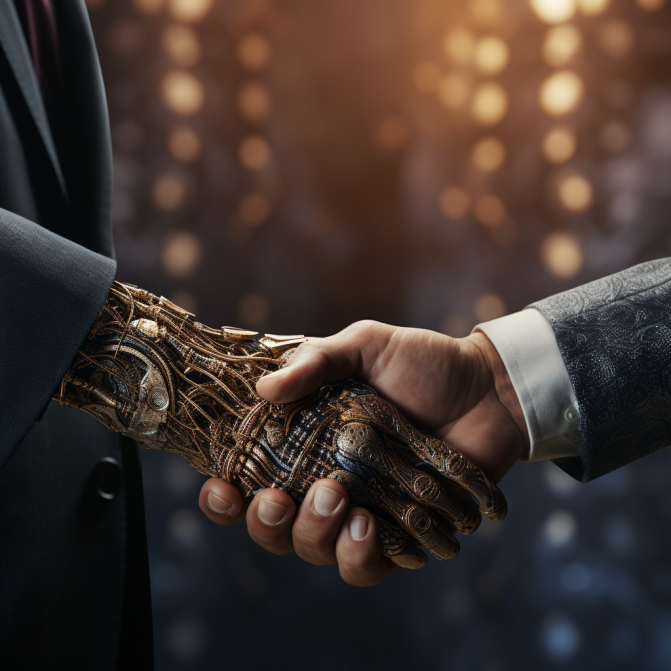
Let’s be honest; it can be a little unsettling when a machine spits out a decision without explanation. It’s like getting advice from a stranger who says, “Trust me,” but won’t tell you why. With responsible AI, it’s a different story.
These systems can explain their reasoning, showing us how they’ve arrived at a particular conclusion based on their analyzed data. This ability to “show its work” helps users feel more confident about the AI’s decisions, leading to greater trust and adoption. After all, it’s easier to trust something when you understand how it works.
Holds AI Development Companies Accountable
Explainable AI holds AI development companies accountable. Some AI systems are ‘black boxes’ because they make decisions without explaining how or why, but with Explainable AI, there’s no room for such mystery. These systems can explain their decision-making process in a clear, understandable way.
If an AI system makes a mistake or shows bias, it’s possible to trace back and understand why it happened. This level of transparency forces AI development companies to be more diligent in their design and training processes, ensuring their models are fair, reliable, and effective. In essence, Explainable AI is like a watchdog, promoting accountability and ethical practices in the AI industry.
Increases Productivity
Explainable AI is a real game-changer when boosting productivity. Here’s how: It’s like having a super-smart, ultra-efficient team member who does their job exceptionally well and explains their process. Because Explainable AI systems can analyze vast amounts of data and make informed decisions quickly, they can significantly speed up tasks, from customer service to business analytics. But the real productivity boost comes from their ability to explain their choices.
This means you don’t have to spend time second-guessing the model. With a clear understanding of AI, teams can incorporate its insights into their strategies and effectively manage their workflows, helping businesses and individuals be more efficient.
Produces Business Value and Value-Generating Properties
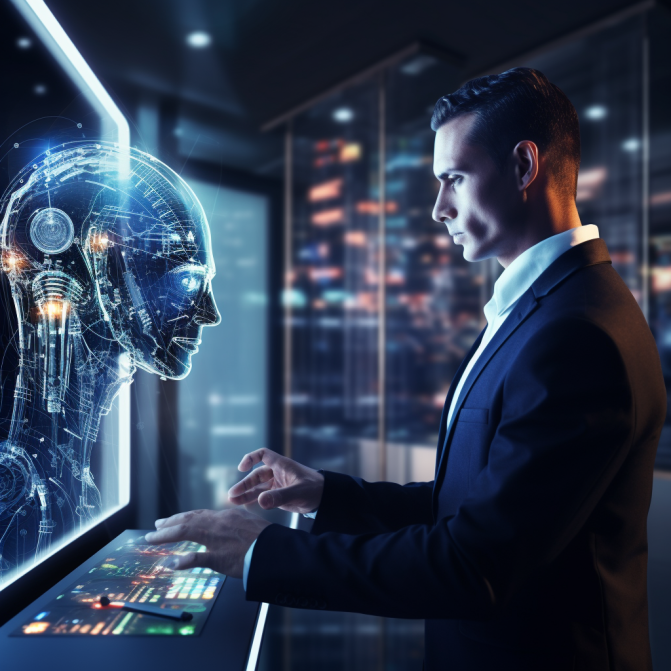
Explainable AI is a powerful tool for generating business value too. It enhances decision-making processes by providing clear, data-driven insights. This means businesses can make more informed decisions, leading to better outcomes and increased profitability. Additionally, XAI improves customer experiences by providing personalized recommendations based on its ability to analyze and learn from data.
But perhaps most importantly, XAI builds trust – a priceless asset in today’s data-driven world. When customers, employees, and stakeholders understand how an AI system makes decisions, they’re more likely to engage with it, leading to stronger relationships and greater business value. When the technical team can explain how an AI system works, the business team can verify that the business is achieving its objectives. Explainable AI is not just a tool for doing business; it’s a strategic asset that can drive AI application growth and success.
Reduces Risks of Using Explainable AI
Explainable AI reduces the risks associated with using AI. Often, the ‘unknown’ is a risk factor – if an AI system makes an incorrect medical diagnosis, and we don’t understand why, it’s impossible to prevent the same error again. XAI changes this by explaining its decisions allowing us to correct any issues with model accuracy and reducing the risk of future mistakes.
Moreover, Explainable AI mitigates reputational and legal risks. If an AI system is unfair, it could damage a company’s reputation or even result in legal action. But with Explainable AI’s transparency, these risks are significantly lessened. In essence, Explainable AI acts like a reliable co-pilot, helping navigate the complexities of AI with greater confidence.
How Can Businesses Make AI More Explainable?
Businesses can make AI more explainable by integrating ethical principles into their operations; employing data ethics in daily routines is essential. This means handling data responsibly, ensuring fairness, and being transparent about how AI models interpret and use this data. Secondly, training is crucial – everyone in the business should understand why explainable AI matter.
Finding the right talent is another critical step. It would be best if you had people who understand AI and can communicate its workings in a clear, relatable way. The use of ‘White Box’ AI technology is the only way to ensure transparency, so businesses must invest in such technology.
AI can identify and correct biases in data or model behavior, reducing the risk of inaccurate outcomes. Lastly, it’s essential to understand what problems Explainable AI solves. Businesses can leverage their capabilities to drive success by knowing what they can do. In essence, making AI more explainable is about integrating transparency, understanding, and ethics into the heart of your AI strategy.
What Can Individuals Do to Adopt Explainable AI Concepts?
Individuals can adopt Explainable AI concepts in several ways. Firstly, investing in training is crucial. Understanding why explainability is essential helps individuals use and trust these systems. There are many resources available, from online courses to webinars, that provide valuable insights.
Secondly, it’s beneficial to learn about explainability technology itself. Knowing how ‘white box’ models work helps individuals understand AI decisions. This knowledge is also good when choosing AI products or services – you’ll want to opt for those prioritizing explainability.
Staying updated on legal and regulatory requirements is another crucial step. The world of AI is constantly evolving, and so are the laws surrounding it. By keeping abreast of these changes, individuals will always use AI in a way that’s ethical, fair, and compliant with the law.
Finally, realizing that adopting Explainable AI practices isn’t just beneficial but necessary to come out on top is critical. In a world where AI is increasingly prevalent, trusting these systems can give individuals an advantage in their personal or professional lives. So, adopting Explainable AI isn’t just about understanding technology – it’s about staying ahead in a rapidly changing world.
Final thoughts
Explainable AI is becoming increasingly crucial for businesses and individuals alike. We can leverage their potential and improve decision-making by making AI systems more transparent, understandable, and trustworthy. Organizations should create a process for risk assessment to evaluate the risks and legal requirements related to explainability for each AI use case. Each use case may have unique concerns, and organizations must address them appropriately.
Individuals should invest in training to better understand why explainable AI matters. Ultimately, Explainable AI is at the heart of responsible AI – it’s a technology that facilitates trust and understanding between humans and machines. Integrating XAI into our routines allows us to better navigate an ever-changing world with greater safety.